Interest in generative AI has led to its widespread adoption across all industries. According to McKinsey’s global survey on AI, 65% of respondents report that their organizations are regularly using generative AI, a figure that has doubled compared to just ten months earlier. This rapid growth highlights the speed of adoption. Generative AI in retail is also experiencing rapid growth, and its implementation across multiple business workflows demonstrates its growing influence in the industry.
Looking deeper into the retail sector, a survey by Salesforce and the Retail AI Council indicates that 36% of retailers are currently using generative AI, and that percentage is projected to reach 45% by 2025. What’s particularly interesting is that 93% of these retailers use generative AI for personalization, such as writing personalized email content and product recommendations. In fact, retailers have started allocating almost 50% of their budget to generative AI to ensure they remain relevant in the market.
This blog takes an in-depth look at the major use cases across retail value chain, real-life examples, and challenges of implementing generative AI in retail. It also explores where retailers can integrate technology right now and what leaders need to know about its capabilities.
What is generative AI and why does it matter for retail?
Generative AI, a subset of artificial intelligence, understands prompts and questions in plain language to produce text or graphics in response. It can analyze massive amounts of data to create personalized content and extract useful insights. Implementing generative AI in retail may seem daunting for entrepreneurs and businesses at first, but the potential benefits outweigh the odds.
Several studies illustrate the positive influence of AI, particularly generative AI in retail:
- A 2024 Nvidia study shows that 69% of retailers reported an increase in annual revenue, while 72% experienced significant cost reductions after implementing AI.
- According to Fortune Business Insights, the global AI-powered retail industry is expected to reach $85.07 billion by 2032.
Retailers can save costs, improve customer service, and increase sales by implementing generative AI into operations. Generative AI not only improves daily operations but also opens new prospects for development and innovation.
With the “what” and “why” of generative AI in retail established, let’s turn our attention to the “how” – how it is being used across the industry.
Use cases of generative AI in retail
A McKinsey survey revealed recently that retailers who have successfully deployed GenAI did one thing right- they did a thorough study of their use cases. This prevented them from wasting their resources on irrelevant areas and incurring losses.
GenAI can impact the entire gamut of retail right from procurement to customer experience.
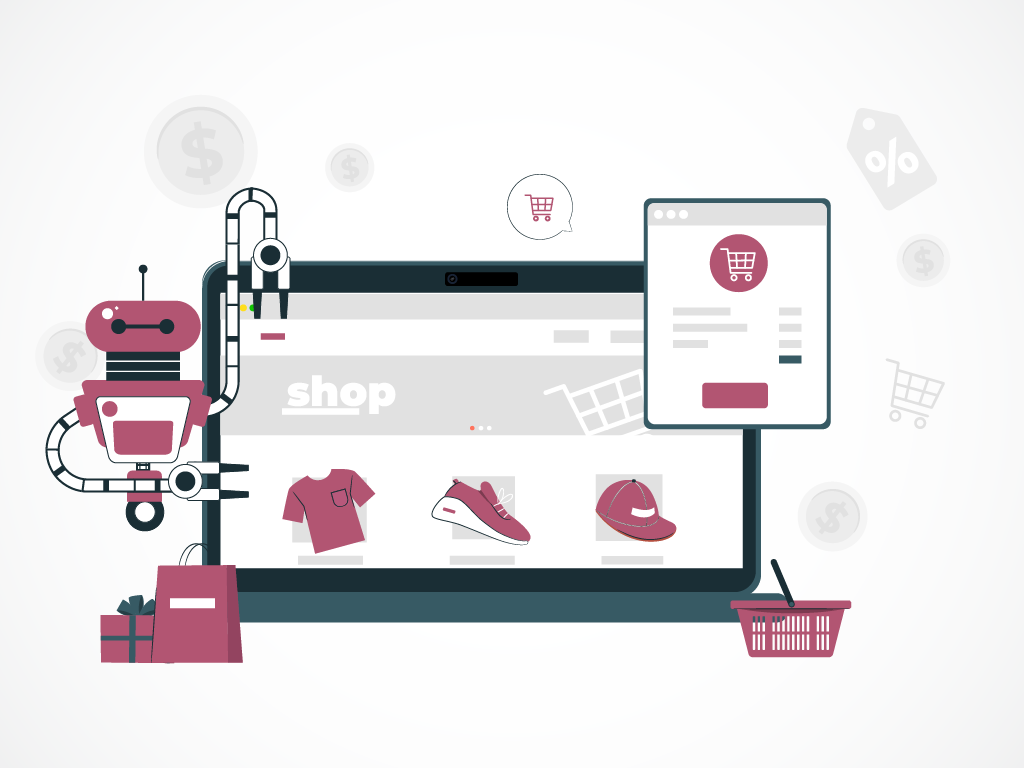
Procurement
For years, retailers have relied on skilled negotiators to get better deals from suppliers. Generative AI (Gen AI) chatbots are giving supplier negotiations a high-tech face-lift. It has changed completely the way procurement teams interact with suppliers. By handling early conversations, GenAI models can reduce wait times and facilitate smoother encounters than before.
But GenAI’s role can be even greater in closing deals. Instead of conducting time-consuming vendor assessments based on limited data, procurement teams can use GenAI to provide comprehensive reports and automated summaries of vendor terms and conditions and other important information. This shift to automation and data-driven insights is helping procurement teams to make better decisions and achieve more successful contract closures.
Distribution
Distribution has long been a source of logistics challenges, particularly around communication and disruption management. Before GenAI, associates managed all interactions with third-party logistics providers, leading to potential communication bottlenecks. Now, GenAI’s chatbots handle initial communication and email messaging, streamlining interactions.
Similarly, responding to disruptions in distribution used to be slow and complex. With GenAI’s support for return management and disruption response, these challenges are being addressed more effectively, leading to faster resolutions and increased operational efficiency.
In-store operations
Generative artificial intelligence (GenAI) automates and simplifies key aspects of in-store retail operations. Previously, employees spent a significant amount of time manually searching for information (pricing, locations, and stock) and interacting with third-party logistics providers.
GenAI-powered assistants now provide instant voice access to critical data, automating information retrieval and significantly reducing reaction times. This automation frees up colleagues from time-consuming tasks, allowing them to focus on more productive activities, such as connecting with customers and making sales.
E-commerce
E-commerce content production and website personalization have always been resource-intensive operations. Previously, creating e-commerce content such as product profiles and descriptions required hundreds of hours of human labor. Website personalization was a manual, rule-based procedure that consumed a lot of the team’s time.
Generative AI (GenAI) is currently disrupting these processes. GenAI has transformed content production from a time-consuming task to an automated process that takes just a few minutes. Even more importantly, GenAI is enabling a shift from static, rule-based personalization to dynamic, spontaneous website personalization through automated front-end development. This real-time, personalized experience enhances customer engagement, fosters loyalty, and drives customer satisfaction, providing e-commerce platforms the competitive edge they need.
Marketing
For years, marketers have struggled with a one-size-fits-all strategy due to limited customer insights drawn primarily from structured data. Generative AI (GenAI) is disrupting this paradigm, ushering in a period of hyper-personalization and increased efficiency. Previously, marketing materials were developed over a lengthy, iterative process.
GenAI now enables marketers to create fully personalized marketing materials for each consumer at unparalleled speed. This is made possible by GenAI’s ability to draw unlimited insights from a variety of unstructured sources, such as product reviews, resulting in a much deeper understanding of consumer preferences and demands.
Back office
Administrative activities in retail back offices used to be slow and inefficient. Before GenAI, functions like HR and payroll were error-prone and required a lot of manual work.
GenAI is now changing these functions with the next generation of lean “white collars.” GenAI-powered chatbots and interfaces, like HR/Finance and software development copilots, are automating administrative tasks, resulting in considerable increases in productivity and accuracy.
Commercial
For years, retailers have used analytics tools in their business operations, but the varying levels of maturity and complexity of these tools have sometimes prevented their widespread adoption.
Generative AI is currently addressing this problem by improving the intuitive interfaces of these tools. This has increased user adoption. In addition to improving usability, GenAI automates creative processes, acting as a “business co-pilot” and allowing retail teams to focus on more strategic efforts.
End-to-end value chain
Understanding the factors that drive retail performance has always been a challenge. Since decisions are often made by individual functions, it can be difficult to identify the true causes of commercial events.
Generative AI (GenAI) is addressing this problem by providing meaningful insights drawn from a diverse set of data. GenAI analyzes both structured data (such as sales transactions) and unstructured data (such as customer reviews and social media posts) to provide a more comprehensive understanding of the business. This helps retailers to make better decisions regarding pricing, promotions, stock allocation, and digital marketing, resulting in better overall performance.
Performance analytics and forecasting
Retailers are inundated with data but turning it into useful insights has always been a struggle. Manual analysis can’t keep pace with the volume and velocity of data collected today, leaving retailers unable to understand the “why” behind their performance.
Generative AI (GenAI) offers a solution by automating data collection and delivering comprehensive, personalized insights in real-time. These insights include root cause analysis, which explains the underlying causes of performance patterns, as well as strategic recommendations that provide a clear path for development. This degree of automation enables continuous performance monitoring, preemptive risk prediction, and rapid plan revisions. Such actions ensure long-term growth and operational efficiency in a competitive retail market.
Customer experience
Gen AI has the potential to increase retail experience by enhancing every stage of the shopping journey for both retailers and customers.
At the idea stage, it can understand customer intent and provide personalized suggestions. For creating a shopping list, AI can generate accurate lists quickly, saving time and effort. When it comes to selecting a retailer, AI can keep customers engaged within the retailer’s ecosystem, offering a seamless experience.
During search and selection of required items, AI can effortlessly navigate catalogs, align product options with customer preferences, and enable smarter basket adjustments with product comparisons based on reviews and trends.
It can simplify order confirmation with conversational preferences, such as “Deliver to my mother’s address except on Wednesdays.” After purchase, AI can offer personalized recommendations, such as how-to videos or guides, to help customers get the most out of their products.
Generative AI can provide retailers with the tools to deliver more personalized, efficient, and intuitive shopping experiences, leading to greater satisfaction and loyalty.
Building on these use cases, let’s look at some real-world examples of how retailers are already using generative AI.
Real-life examples of Generative AI implementation in retail
Application Area | Example | Benefit/Outcome |
Product Descriptions & Content Creation | Amazon, Shopify: Automatically generate customized product descriptions leveraging item attributes, customer demographics, and purchasing behavior. | Improved discoverability and resonance with target audiences. |
Virtual Try-Ons & Styling Recommendations | Nike, Sephora: Power virtual try-on tools for products like shoes, makeup, and clothing. AI-driven styling assistants suggest outfits and complementary products. | Enhanced customer experience, increased purchase confidence, personalized styling advice. |
Chatbots & Personalized Assistance | H&M, IKEA: Deploy AI chatbots for instant customer support, answering queries, guiding purchases, and recommending personalized products. | Streamlined customer interactions, enhanced shopping experience, personalized product recommendations, improved customer service efficiency. |
Dynamic Pricing & Promotions | Walmart: Utilize AI algorithms to adjust prices in real time based on market trends, competitor pricing, and customer demand. | Maximized sales, optimized profit margins, improved customer satisfaction through competitive pricing. |
Marketing Enhancements | Amazon: Launched an AI-powered image generation tool to transform basic product photos into realistic lifestyle images (e.g., placing a sofa in a virtual living room). | Improved ad click-through rates (up to 40%), enhanced product visualization, improved customer engagement. |
Software Development Productivity | Mercado Libre: Deployed generative AI “copilots” to automate repetitive tasks and provide starting points for complex work. | Increased developer productivity and satisfaction, reduced time spent on engineering tasks (by up to 60%), allowing teams to focus on higher-value work. |
In-Store Operations | Lindex: Introduced “Lindex Copilot,” a generative AI tool trained on sales and store data, providing employees with personalized advice, daily task guidance, and insights. | Improved operational efficiency, enhanced decision-making on the shop floor, personalized guidance for store employees. |
Supply Chain Optimization | Target: Use generative AI to forecast demand, manage stock, and streamline logistics. | Improved demand forecasting, optimized stock management, streamlined logistics, ensured timely deliveries, reduced costs, enhanced supply chain reliability. |
Steps to implement generative AI in retail
Even with all these use cases looming large, the question still persists, “How can retailers successfully use generative AI to disrupt their operations?” While the possibilities are endless, a strategic, phased approach is required to avoid typical pitfalls. Focusing on high-value use cases and developing a clear implementation plan is critical.
The following sections provide a strategy for successfully implementing generative AI in retail.
Data acquisition
For generative AI to produce accurate results, high-quality data is needed. It enables AI to provide personalized content, make correct suggestions, and offer efficient predictive solutions.
- Data collection: Collect information about consumers, products, and transactions from a variety of sources, including e-commerce sites, social media, and in-store encounters. For activities like content generation or chatbot responses, generative AI may also require unstructured data, such as customer reviews, photos, or text input.
- Data validation and cleanup: Clean and verify data to confirm its accuracy. Remove duplications, correct inconsistencies, and standardize formats to increase the quality of AI results.
- Data exploration: Conduct exploratory analysis of the data to uncover trends or gaps. This stage confirms that the data is consistent with the retail challenges that generative AI is designed to address, such as personalized product suggestions or automated marketing materials.
Model development
Generative AI models are designed to provide outputs such as product descriptions, marketing campaigns, and artwork. Developing these models requires certain steps:
- Model selection: Select the appropriate generative AI models, such as GPT for text creation or GANs (generative adversarial networks) to produce visual assets such as photographs and product designs.
- Model training: Use retail-specific data such as product catalogues, customer preferences and past marketing content to train the model to produce relevant, high-quality results.
- Model fine-tuning: Customize pre-trained generative AI models (such as OpenAI’s GPT or similar) to meet specific retail requirements. Fine-tuning ensures that the model generates contextually appropriate results while adhering to brand tone and requirements.
- Evaluation: Evaluate model outputs for quality, consistency, and relevance. For example, check produced product descriptions or chatbot responses to verify that they align with consumer expectations and business goals.
Deployment
Once verified, generative AI models can be deployed across retail operations to assist with automation and decision-making.
- Workflow integration: Integrate generative AI into platforms such as e-commerce websites, marketing tools, and CRM systems. This can include incorporating AI-powered chatbots for customer service or email marketing automation.
- Testing: To ensure smooth operation, simulate real-world circumstances before deploying on a larger scale. Test dynamic pricing models, for example during seasonal promotions, or AI-generated product suggestions during peak traffic periods.
Monitoring and optimization
Ongoing monitoring is required to ensure generative AI remains effective and aligned with business goals.
- Performance tracking: Track the impact of AI on important KPIs such as conversion rates, customer engagement, and inventory turnover.
- Regular updates: Use new data to improve the accuracy and relevance of the model over time. For example, fine-tune AI using current sales data or consumer feedback.
- Feedback loops: Collect feedback from both staff and consumers to uncover areas where AI can improve. This ensures the system adapts to changing retail demands.
Even with a well-defined implementation plan, retailers must be prepared for diverse obstacles that may arise. Let’s look at some of the major challenges.
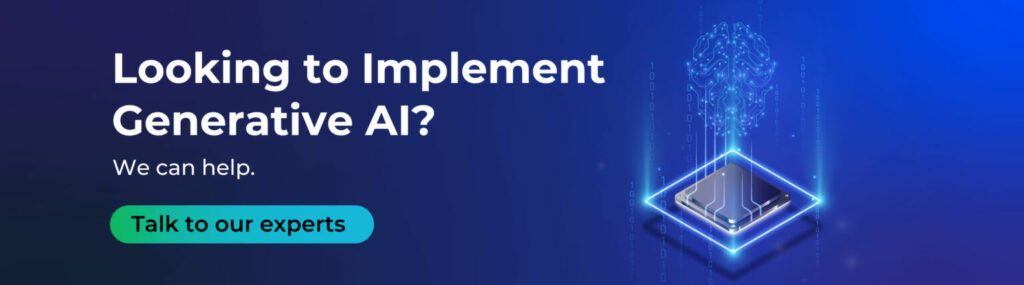
Challenges in implementing generative AI in retail
According to a global consumer survey, consumers are highly intrigued by the promise of generative AI in retail, with 87% of those who have used it expressing enthusiasm for its capabilities. However, realizing this promise and efficiently integrating generative AI into existing retail procedures and consumer experiences poses significant challenges.
Let’s look at the challenges that arise with implementing generative AI in retail and how to solve them.
Data quality
Poor quality data can lead to inaccurate opinions and information, which dramatically reduces the usefulness of AI systems. This notion is often summed up by the expression “garbage in, garbage out,” meaning that if faulty or erroneous data is fed into an AI system, the results will be unreliable.
High-quality, well-structured data is needed to ensure that AI models produce accurate and relevant results, as errors in data can propagate through systems and have significant effects on decision-making.
How to minimize it: Retailers can manage this by implementing robust data governance practices, using data cleansing tools to eliminate errors, and conducting frequent audits to ensure data accuracy and integrity.
These processes ensure that AI systems only get high-quality, reliable data, resulting in accurate and actionable insights for better decision-making.
Bias in AI models
AI systems can replicate human biases if they are trained with biased data or built without taking into account diversity and inclusion. For example, a study by the NHTSA showed that women are 17% more likely than men to die in traffic accidents, despite being better drivers.
This gap arises because female dummies are frequently excluded from crash tests or placed exclusively in the passenger seat. By providing a broad collection of data, AI models can minimize bias and increase their ability to successfully generalize.
To mitigate this: Retailers should carefully select training data, diversify their AI development teams, and conduct frequent bias audits to detect and resolve any flaws. They should also include ethical rules when designing AI systems to promote fairness and transparency.
Integrating AI into existing systems
Integrating generative AI into existing retail systems, such as e-commerce platforms and supply chain management tools, can be challenging and expensive. Retailers may encounter issues such as ensuring data interoperability, resolving security issues, and managing any disruption to current procedures.
They must also consider the ongoing expenses of maintaining, updating, and monitoring AI systems to ensure optimal performance and data compliance.
How to minimize it: To successfully incorporate generative AI into retail systems, retailers should first conduct a thorough audit of their current infrastructure to identify compatibility gaps. Partnering with experienced AI vendors or AI experts can help ensure easy integration while also addressing security concerns through strong encryption and compliance procedures.
Retailers can also use change management strategies to reduce workflow disruptions, such as staff training and phased rollouts. Additionally, budgeting for ongoing expenses such as system maintenance, upgrades, and monitoring will ensure AI systems operate efficiently and comply with data standards.
Issues with staff training and change management
Getting employees to use generative AI technologies and adapt to change isn’t always straightforward, especially for non-technical teammates.
How to address it: Retailers can help by providing frequent training sessions, talking clearly about how AI could improve work, and bringing staff on board early in the process. This makes everyone feel involved and fosters a sense of ownership.
Talent gap
A lack of competent personnel to design, implement, and manage AI systems is a serious impediment. This skills gap impedes innovation, makes it difficult to find the right people, and increases recruitment costs.
Companies struggle to fill positions in areas such as machine learning, data science, and AI infrastructure, leading to project delays and bottlenecks.
How to minimize it: Retailers can address this issue by working with external AI vendors or AI experts to quickly gain the knowledge they need and close skills gaps.
Partnering with universities and innovation hubs is another great option to connect with emerging talent and develop long-term partnerships with competent workers. These methods can help companies accelerate their AI initiatives and capitalize on this revolutionary technology.
Data privacy concerns
Applying generative AI in retail raises concerns about data privacy and security, especially when collecting and processing sensitive personal information (PII). Protecting PII, such as names, addresses, and payment information, is critical to maintaining consumer trust and complying with data protection regulations.
How to minimize it: Retailers must follow strict data privacy standards and invest in secure data storage and management solutions. They should also be transparent about their data policies and seek customer approval before collecting any personal information.
To ensure compliance, they can use strong encryption, strict access restrictions, frequent security audits, and keep up with legislative regulations such as GDPR or CCPA.
Scaling generative AI in retail
While early experiments with generative AI are yielding considerable benefits, retailers must prioritize growing their AI operations to fully realize the technology’s promise. To do so, retail executives must focus on five critical imperatives:
Identify key area
Start by assessing your organization’s key domains to determine which areas would benefit most from change. Focus on processes, techniques, or technologies that can deliver significant value by improving or updating.
Upskill talent
Invest in the training and development of your team to ensure they are prepared to adapt to new technologies and procedures. Workshops, certificates and on-the-job training can help fill gaps.
Create a centralized, cross-functional team
Create a dedicated team comprised of people from various departments to ensure diversity of knowledge and opinions. This team will streamline communication and oversee the change process, ensuring that activities are aligned with company goals
Leverage external partners
Bring in experienced external partners or consultants who can provide knowledge, tools, or resources that your internal team may lack. Collaborating with external experts can accelerate transformation and mitigate risks.
Measure impact
Establish explicit metrics to measure the effectiveness of transformation efforts. Monitor and evaluate this data periodically to ensure progress and make necessary modifications to optimize the impact.
Conclusion
In the coming years, generative AI in retail is poised to play a significant role in shaping future e-commerce experiences. Regardless of whether retailers choose to implement this technology immediately, it’s critical to stay informed and keep an open mind. This will allow retailers to be prepared to capitalize on generative AI’s potential and maintain their lead in a volatile and competitive business.
Want to explore how generative AI can help your business? Get in touch with us now!
FAQs
How can generative ai in retail improve customer experience?
Generative AI in retail can personalize shopping experiences by evaluating user data and adjusting product suggestions. It can also generate dynamic content, such as personalized emails or ads, to ensure communications are relevant and entertaining for each consumer.
What are some practical applications of generative ai in retail operations?
Generative AI in retail can improve inventory management by forecasting demand patterns and optimizing stock levels. It can also help produce product descriptions, design visual material for marketing campaigns, and even model store layouts to improve efficiency and consumer flow.